Sitemap
A list of all the posts and pages found on the site. For you robots out there is an XML version available for digesting as well.
Pages
Posts
Future Blog Post
Published:
This post will show up by default. To disable scheduling of future posts, edit config.yml
and set future: false
.
Blog Post number 4
Published:
This is a sample blog post. Lorem ipsum I can’t remember the rest of lorem ipsum and don’t have an internet connection right now. Testing testing testing this blog post. Blog posts are cool.
Blog Post number 3
Published:
This is a sample blog post. Lorem ipsum I can’t remember the rest of lorem ipsum and don’t have an internet connection right now. Testing testing testing this blog post. Blog posts are cool.
Blog Post number 2
Published:
This is a sample blog post. Lorem ipsum I can’t remember the rest of lorem ipsum and don’t have an internet connection right now. Testing testing testing this blog post. Blog posts are cool.
Blog Post number 1
Published:
This is a sample blog post. Lorem ipsum I can’t remember the rest of lorem ipsum and don’t have an internet connection right now. Testing testing testing this blog post. Blog posts are cool.
portfolio
Portfolio item number 1
Short description of portfolio item number 1
Portfolio item number 2
Short description of portfolio item number 2
publications
A Neuro-Symbolic Classifier with Optimized Satisfiability for Monitoring Security Alerts in Network Traffic
Published in Applied Sciences, MDPI, 2022
We introduce in this paper a neuro-symbolic predictive model based on Logic Tensor Networks, capable of discriminating and at the same time of explaining the bad connections, called alerts or attacks, and the normal connections. The proposed classifier incorporates both the ability of deep neural networks to improve on their own through learning from experience and the interpretability of the results provided by the symbolic artificial intelligence approach. Compared to other existing solutions, we advance in the discovery of potential security breaches from a cognitive perspective. By introducing the reasoning in the model, our aim is to further reduce the human staff needed to deal with the cyber-threat hunting problem. To justify the need for shifting towards hybrid systems for this task, the design, the implementation, and the comparison of the dense neural network and the neuro-symbolic model is performed in detail. While in terms of standard accuracy, both models demonstrated similar precision, we further introduced for our model the concept of interactive accuracy as a way of querying the model results at any time coupled with deductive reasoning over data. By applying our model on the CIC-IDS2017 dataset, we reached an accuracy of 0.95, with levels of satisfiability around 0.85. Other advantages such as overfitting mitigation and scalability issues are also presented.
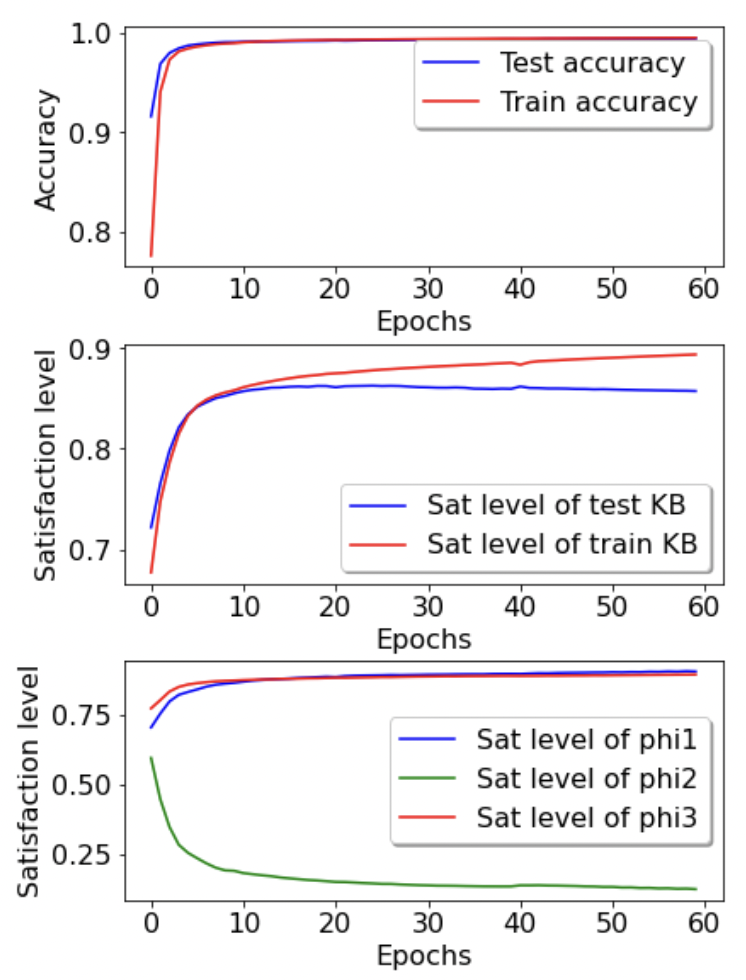
Recommended citation:
@article{onchis2022neuro, title={A Neuro-Symbolic Classifier with Optimized Satisfiability for Monitoring Security Alerts in Network Traffic}, author={Onchis, Darian and Istin, Codruta and Hogea, Eduard}, journal={Applied Sciences}, volume={12}, number={22}, pages={11502}, year={2022}, publisher={MDPI} }
Download Paper
Advantages of a neuro-symbolic solution for monitoring IT infrastructures alerts
Published in SYNASC, IEEE, 2022
The classification and at the same time the inter-active characterization of both bad connections, called alerts or attacks, as well as normal connections, is a must for monitoring network traffic. For this specific task, we developed in this study a neuro-symbolic predictive model based on Logic Tensor Networks. Moreover, we present in detail the advantages and disadvantages of using our hybrid system versus the usage of a standard feed-forward deep neural network classifier. For a relevant comparison, the same dataset was used during training and the metrics resulted have been compared. An overview shows that while both algorithms have similar precision, the hybrid approach gives also the possibility to have interactive explanations and deductive reasoning over data.
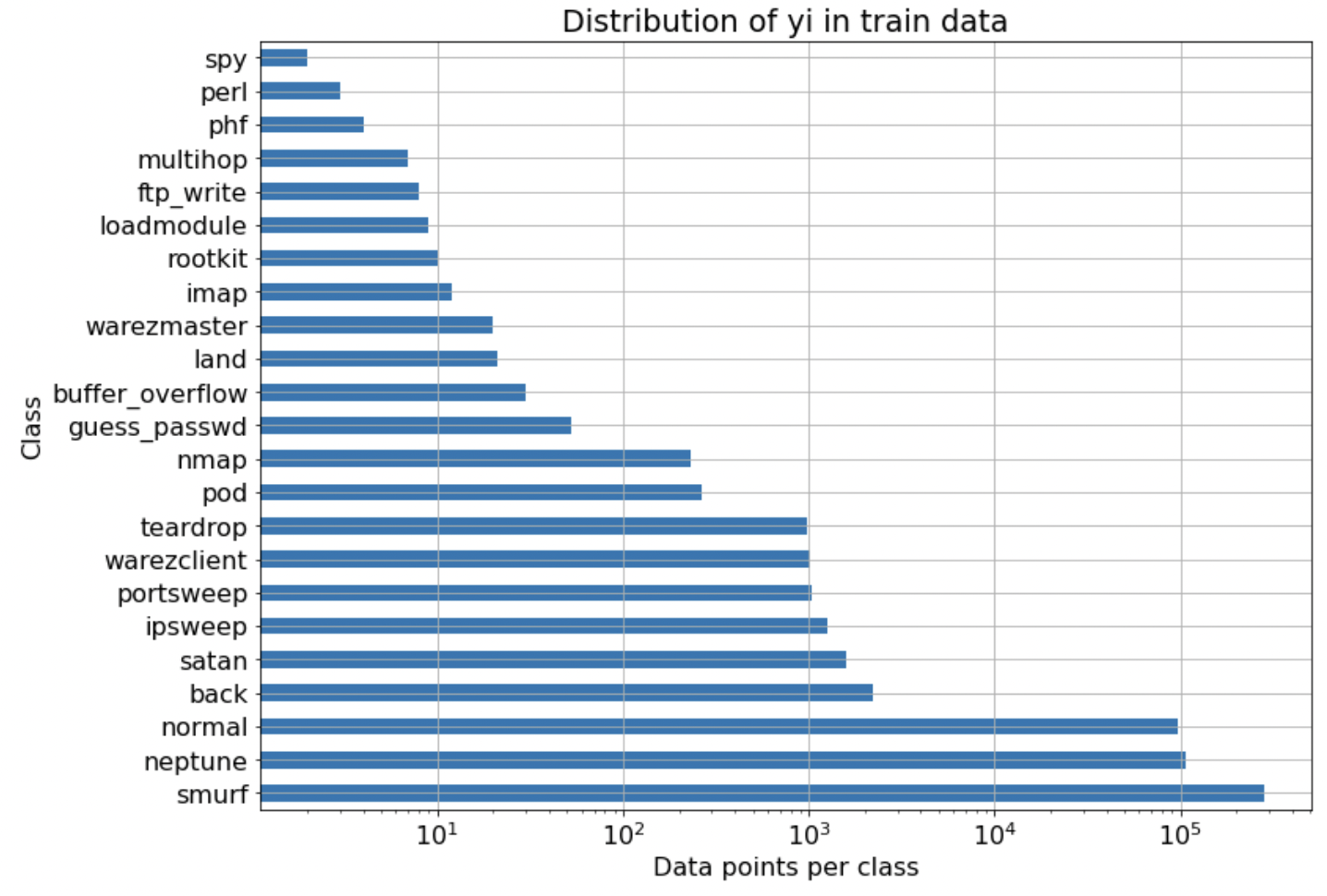
Recommended citation:
@inproceedings{onchis2022advantages, title={Advantages of a neuro-symbolic solution for monitoring IT infrastructures alerts}, author={Onchis, Darian M and Istin, Codruta and Eduard-Florin, Hogea}, booktitle={2022 24th International Symposium on Symbolic and Numeric Algorithms for Scientific Computing (SYNASC)}, pages={189--194}, year={2022}, organization={IEEE} }
Download Paper
Neuro-symbolic model for cantilever beams damage detection
Published in Computers in Industry, Elsevier, 2023
In the last decade, damage detection approaches swiftly changed from advanced signal processing methods to machine learning and especially deep learning models, to accurately and non-intrusively estimate the state of the beam structures. But as the deep learning models reached their peak performances, also their limitations in applicability and vulnerabilities were observed. One of the most important reason for the lack of trustworthiness in operational conditions is the absence of intrinsic explainability of the deep learning system, due to the encoding of the knowledge in tensor values and without the inclusion of logical constraints. In this paper, we propose a neuro-symbolic model for the detection of damages in cantilever beams based on a novel cognitive architecture in which we join the processing power of convolutional networks with the interactive control offered by queries realized through the inclusion …
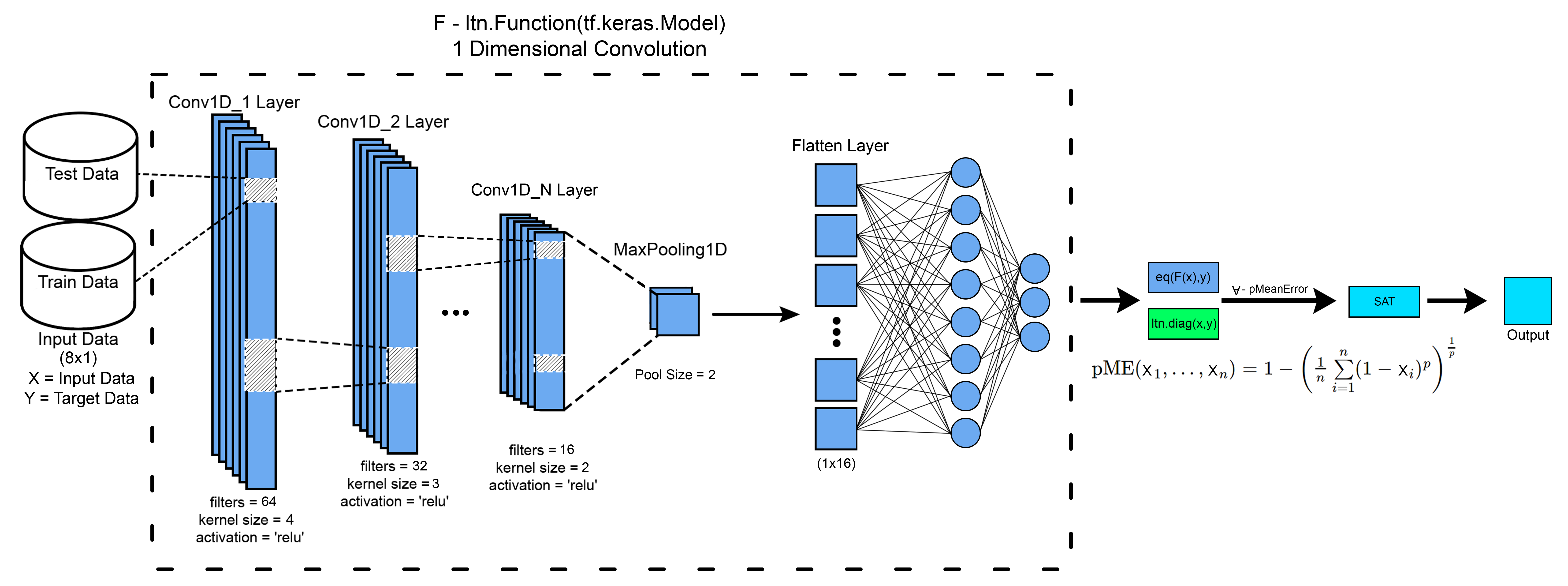
Recommended citation:
@article{onchis2023neuro, title={Neuro-symbolic model for cantilever beams damage detection}, author={Onchis, Darian M and Gillich, Gilbert-Rainer and Hogea, Eduard and Tufisi, Cristian}, journal={Computers in Industry}, volume={151}, pages={103991}, year={2023}, publisher={Elsevier} }
Download Paper
Exemplar-Free Feature Selection via Guided Translation and Optimization Towards Robust Class-Incremental Learning
Published in Unknown Venue, 2024
Being able to constantly retrain a model with new data, while not retaining the old one, and still being able to successfully classify both is an interesting concept that has seen a surge in interest in both academia and industry. This specific field, called Exemplarfree class-incremental learning (EFCIL) poses significant challenges, primarily due to catastrophic forgetting, necessitating a delicate balance between stability and plasticity to accurately recognize both new and previous classes. Traditional EFCIL approaches typically skew towards either model plasticity through successive fine-tuning or stability by employing a fixed feature extractor beyond the initial incremental state. Building upon the foundational FeTrIL framework, our research extends into novel experimental domains to examine the efficacy of various oversampling techniques and dynamic optimization strategies across multiple challenging datasets and incremental settings. We specifically explore how oversampling impacts accuracy relative to feature availability and how different optimization methodologies, including dynamic recalibration and feature pool diversification, influence incremental learning outcomes. The results from these comprehensive experiments, conducted on CIFAR100, Tiny-ImageNet, and an ImageNet-Subset, underscore the superior performance of FeTrIL++ in balancing accuracy for both new and past classes against ten contemporary methods. Notably, our extensions reveal the nuanced impacts of oversampling and optimization on EFCIL, contributing to a more refined understanding of feature-space manipulation for class incremental learning. FeTrIL and …
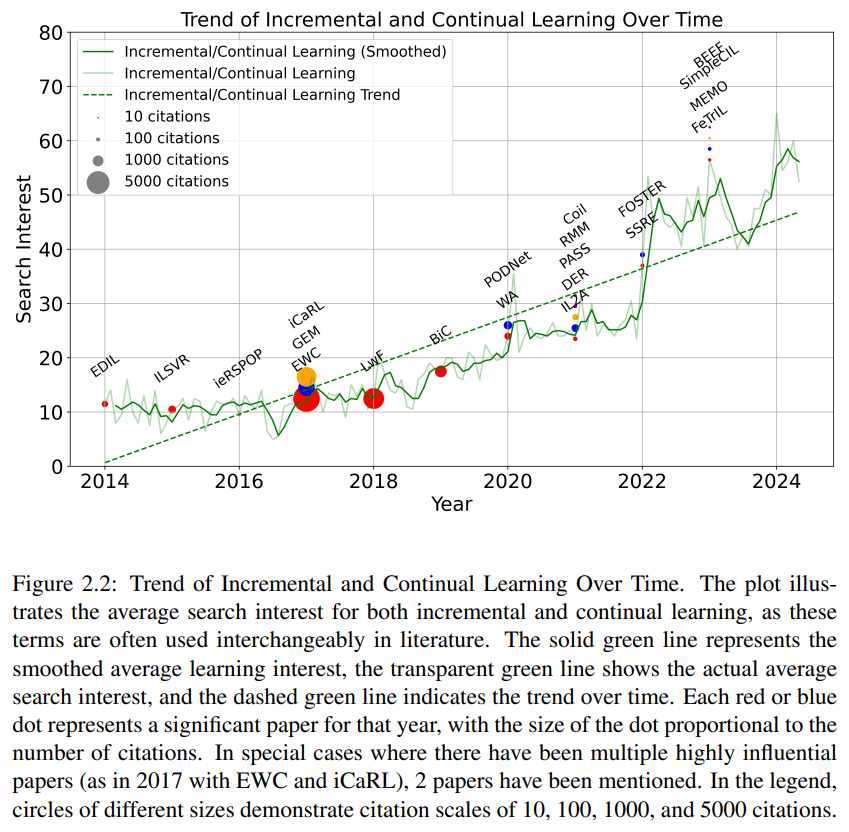
Recommended citation:
@article{onchisexemplar, title={Exemplar-Free Feature Selection via Guided Translation and Optimization: Towards Robust Class-Incremental Learning}, author={Onchis, Darian and Hogea, Eduard-Florin} }
Download Paper | Download Slides
FeTrIL++ Feature Translation for Exemplar-Free Class-Incremental Learning with Hill-Climbing
Published in arXiv, 2024
Exemplar-free class-incremental learning (EFCIL) poses significant challenges, primarily due to catastrophic forgetting, necessitating a delicate balance between stability and plasticity to accurately recognize both new and previous classes. Traditional EFCIL approaches typically skew towards either model plasticity through successive fine-tuning or stability by employing a fixed feature extractor beyond the initial incremental state. Building upon the foundational FeTrIL framework, our research extends into novel experimental domains to examine the efficacy of various oversampling techniques and dynamic optimization strategies across multiple challenging datasets and incremental settings. We specifically explore how oversampling impacts accuracy relative to feature availability and how different optimization methodologies, including dynamic recalibration and feature pool diversification, influence incremental learning outcomes. The results from these comprehensive experiments, conducted on CIFAR100, Tiny-ImageNet, and an ImageNet-Subset, under-score the superior performance of FeTrIL in balancing accuracy for both new and past classes against ten contemporary methods. Notably, our extensions reveal the nuanced impacts of oversampling and optimization on EFCIL, contributing to a more refined understanding of feature-space manipulation for class incremental learning. FeTrIL and its extended analysis in this paper FeTrIL++ pave the way for more adaptable and efficient EFCIL methodologies, promising significant improvements in handling catastrophic forgetting without the need for exemplars.
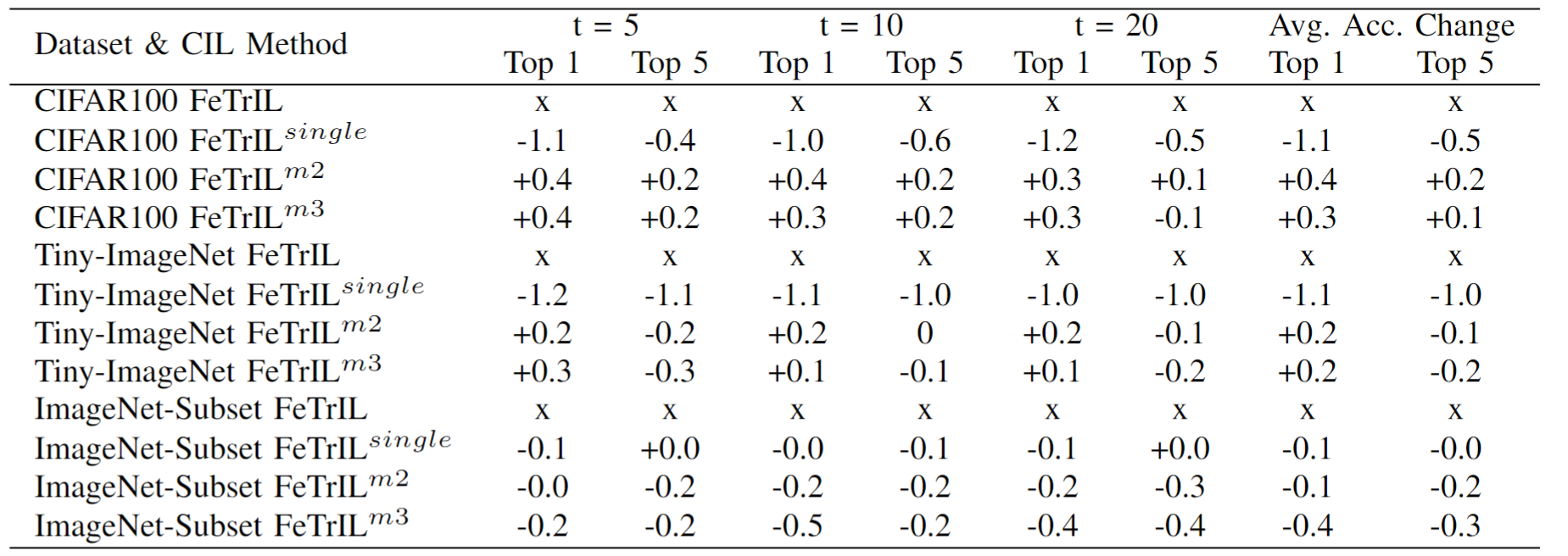
Recommended citation:
@article{hogea2024fetril++, title={FeTrIL++: Feature Translation for Exemplar-Free Class-Incremental Learning with Hill-Climbing}, author={Hogea, Eduard and Popescu, Adrian and Onchis, Darian and Petit, Gregoire}, journal={arXiv preprint arXiv:2403.07406}, year={2024} }
Download Paper
Predictive Modeling for Diabetes Using GraphLIME
Published in medRxiv, 2024
The purpose of this paper is to present a detailed investigation of the advantages of employing GraphLIME (Local Interpretable Model Explanations for Graph Neural Networks) for the trustworthy prediction of diabetes mellitus. Our pursuit involves identifying the strengths of GraphLIME combined with the attention-mechanism over the standard coupling of deep learning neural networks with the original LIME method. The system build this way, provided us a proficient method for extracting the most relevant features and applying the attention mechanism exclusively to those features. We have closely monitored the performance metrics of the two approaches and conducted a comparative analysis. Leveraging attention mechanisms, we have achieved an accuracy of 92.6% for the addressed problem. The model’s performance is meticulously demonstrated throughout the study, and the results are furthermore evaluated using the Receiver Operating Characteristic (ROC) curve. By implementing this technique on a dataset of 768 patients diagnosed with or without diabetes mellitus, we have successfully boosted the model’s performance by over 18%.
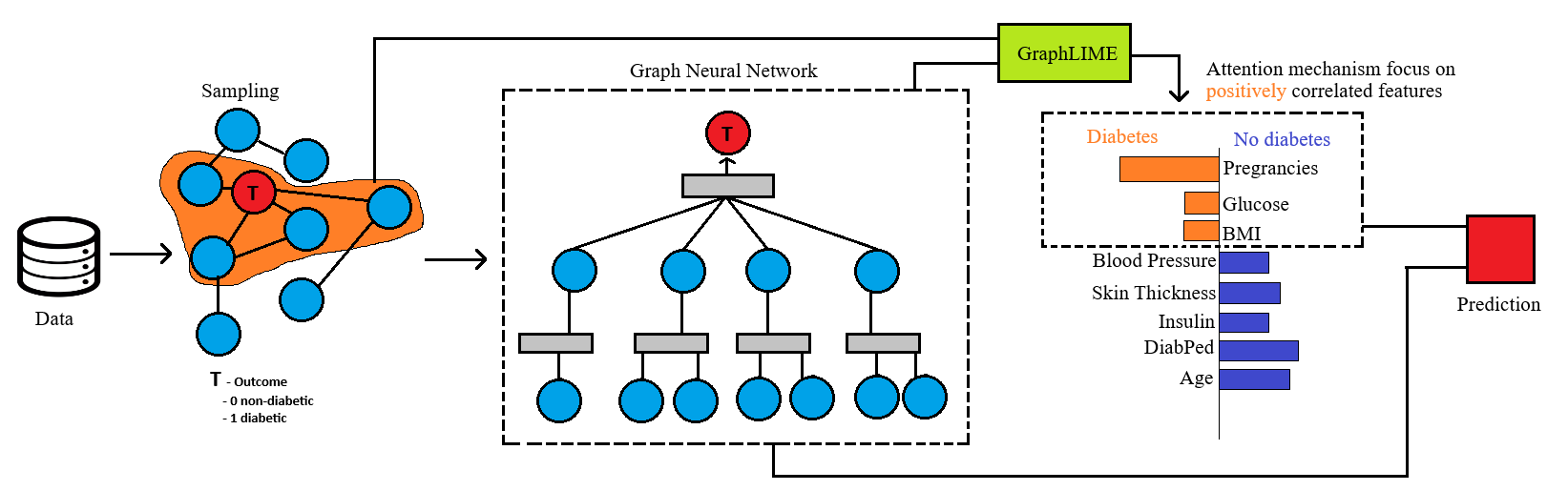
Recommended citation:
@article{costi2024predictive, title={Predictive Modeling for Diabetes Using GraphLIME}, author={Costi, Flavia and Onchis, Darian and Hogea, Eduard and Istin, Codruta}, journal={medRxiv}, pages={2024--03}, year={2024}, publisher={Cold Spring Harbor Laboratory Press} }
Download Paper
Sisteme Hibride de Invatare Automata si Aplicatii
Published in Unknown Venue, 2024
In this paper, a deep neural network approach and a neuro-symbolic one are proposed for classification and regression. The neuro-symbolic predictive models based on Logic Tensor Networks are capable of discriminating and in the same time of explaining the characterization of bad connections, called alerts or attacks, and of normal connections. The proposed hybrid systems incorporate both the ability of deep neural networks to improve on their own through experience and the interpretability of the results provided by symbolic artificial intelligence approach. To justify the need for shifting towards hybrid systems, explanation, implementation, and comparison of the dense neural network and the neuro-symbolic network is performed in detail. For the comparison to be relevant, the same datasets were used in training and the metrics resulted have been compared. A review of the resulted metrics shows that while both methods have similar precision in their predictive models, with Logic Tensor Networks being also possible to have interactive accuracy and deductive reasoning over data. Other advantages and disadvantages such as overfitting mitigation and scalability issues are also further discussed.

Recommended citation:
@article{EduardHogeaandDarianOnchis2024, author = {Eduard Hogea and Darian Onchis}, title = {Sisteme Hibride de Invatare Automata si Aplicatii}, journal = {Unknown Venue}, year = {2024} }
Download Paper | Download Slides
The ethical situation of DALL-E 2
Published in arXiv, 2024
A hot topic of Artificial Intelligence right now is image generation from prompts. DALL-E 2 is one of the biggest names in this domain, as it allows people to create images from simple text inputs, to even more complicated ones. The company that made this possible, OpenAI, has assured everyone that visited their website that their mission is to ensure that artificial general intelligence benefits all humanity. A noble idea in our opinion, that also stood as the motive behind us choosing this subject. This paper analyzes the ethical implications of an AI image generative system, with an emphasis on how society is responding to it, how it probably will and how it should if all the right measures are taken.
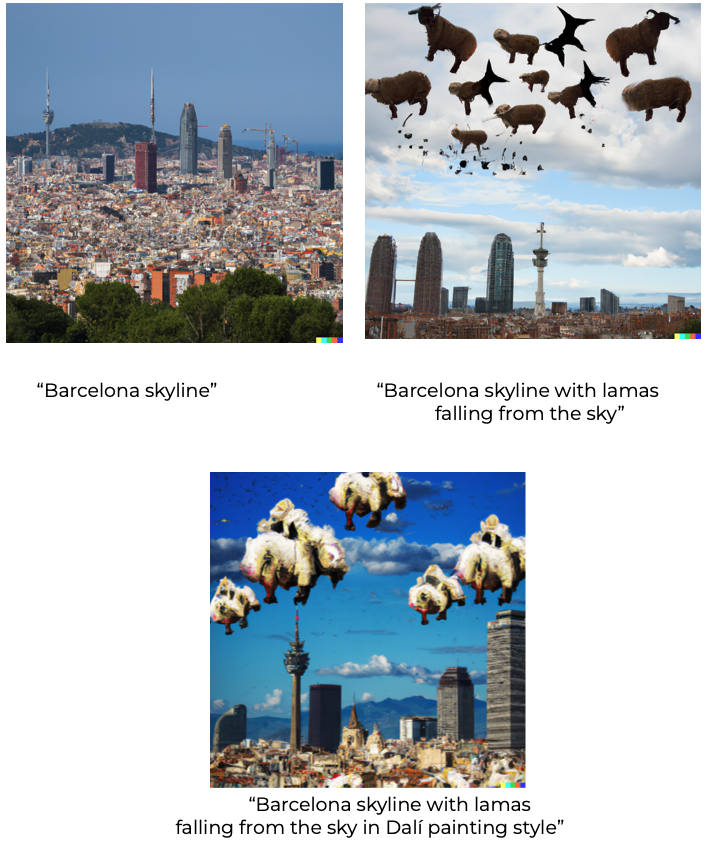
Recommended citation:
@article{hogea2024ethical, title={The ethical situation of DALL-E 2}, author={Hogea, Eduard and Rocafortf, Josem}, journal={arXiv preprint arXiv:2405.19176}, year={2024} }
Download Paper
What Kills Our Mind? Unveiling the Mysteries with Longitudinal Insights from the OASIS Dataset Study
Published in ResearchGate, 2024
In this research project, I undertake a comprehensive longitudinal analysis of the OASIS MRI/Alzheimer’s dataset, an extensive collection of data from 150 subjects aged 60 to 96, including 373 imaging sessions. The dataset encompasses multiple T1-weighted MRI scans for each subject, spanning various visits, and includes variables such as demographics, clinical information, and derived anatomic volumes. The study’s core objective is to analyze the progression of dementia, with a particular focus on Alzheimer’s disease. Key indicators such as Mini-Mental State Examination (MMSE) scores and Clinical Dementia Rating (CDR) are used to evaluate cognitive impairment and dementia severity. The analysis made in such a way to explore correlations between dementia progression and factors like age, education, socioeconomic status, and brain volume metrics. A pivotal aspect of this research is the comparison between two statistical methodologies: the Random Coefficients Model and the Mean Response Model. Employing Generalized Linear Mixed Models (GLMM) and Ordinal Logistic Regression, these models are chosen for their aptness in handling the longitudinal and categorical nature of the data. The research methodology includes careful handling of missing values, scaling of continuous predictors, and computation of correlation matrices. This study aims to elucidate patterns in dementia progression, with a focus on the efficacy of each model in interpreting the dynamic changes over time in a longitudinal dataset. The results section presents detailed findings, highlighting the comparative performance of the two models in capturing the complexities of Alzheimer’s disease progression. The Random Coefficients model outperforms the Mean Response one, considering the evaluation metrics used.
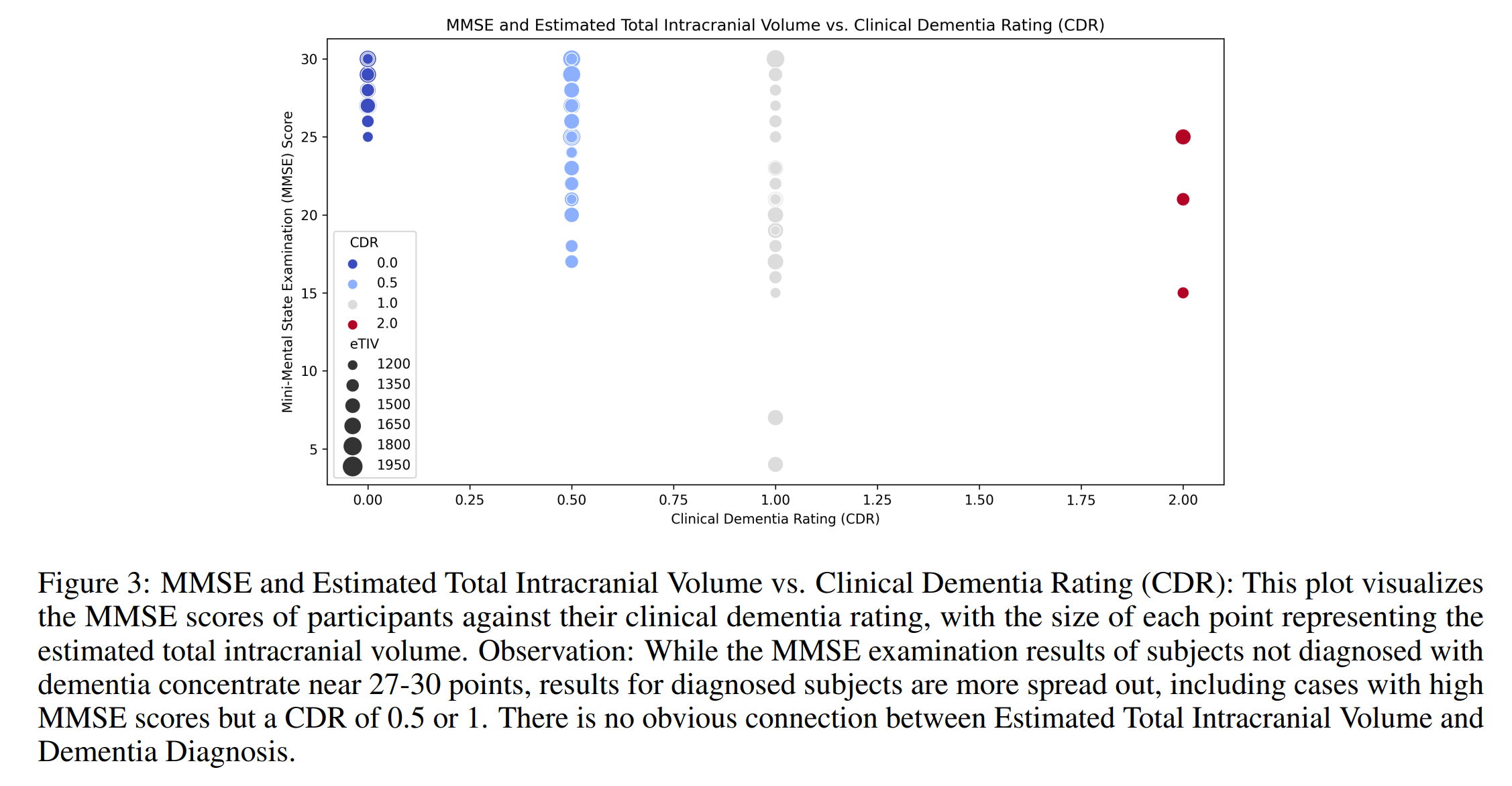
Recommended citation:
@article{hogeakills, title={WHAT KILLS OUR MIND? UNVEILING THE MYSTERIES WITH LONGITUDINAL INSIGHTS FROM THE OASIS DATASET STUDY}, author={Hogea, Eduard} }
Download Paper | Download Slides
Enhanced Anomaly Detection in Automotive Systems Using SAAD Statistical Aggregated Anomaly Detection
Published in arXiv/SYNASC(future), 2024
This paper presents a novel anomaly detection methodology termed Statistical Aggregated Anomaly Detection (SAAD). The SAAD approach integrates advanced statistical techniques with machine learning, and its efficacy is demonstrated through validation on real sensor data from a Hardware-in-the-Loop (HIL) environment within the automotive domain. The key innovation of SAAD lies in its ability to significantly enhance the accuracy and robustness of anomaly detection when combined with Fully Connected Networks (FCNs) augmented by dropout layers. Comprehensive experimental evaluations indicate that the standalone statistical method achieves an accuracy of 72.1%, whereas the deep learning model alone attains an accuracy of 71.5%. In contrast, the aggregated method achieves a superior accuracy of 88.3% and an F1 score of 0.921, thereby outperforming the individual models. These results underscore the effectiveness of SAAD, demonstrating its potential for broad application in various domains, including automotive systems.
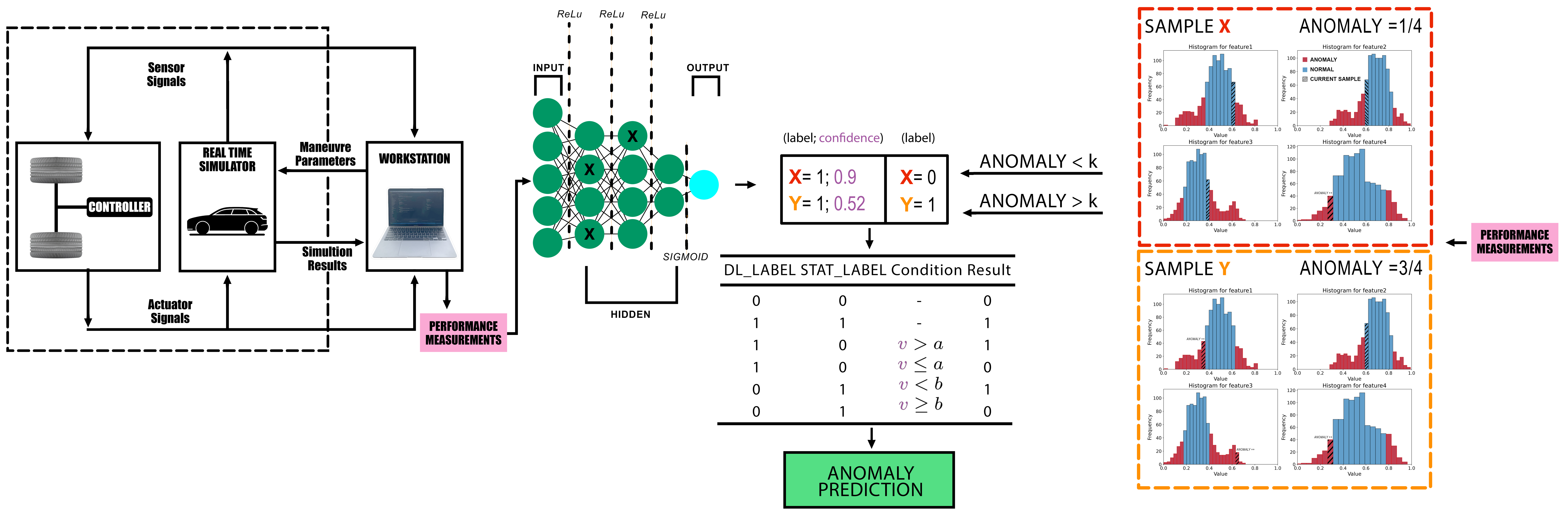
Recommended citation:
@article{goina2024enhanced, title={Enhanced Anomaly Detection in Automotive Systems Using SAAD: Statistical Aggregated Anomaly Detection}, author={Goina, Dacian and Hogea, Eduard and Maties, George}, journal={arXiv preprint arXiv:2406.08516}, year={2024} }
Download Paper
talks
Talk 1 on Relevant Topic in Your Field
Published:
This is a description of your talk, which is a markdown files that can be all markdown-ified like any other post. Yay markdown!
Conference Proceeding talk 3 on Relevant Topic in Your Field
Published:
This is a description of your conference proceedings talk, note the different field in type. You can put anything in this field.
teaching
Teaching experience 1
Undergraduate course, University 1, Department, 2014
This is a description of a teaching experience. You can use markdown like any other post.
Teaching experience 2
Workshop, University 1, Department, 2015
This is a description of a teaching experience. You can use markdown like any other post.